New research published in January’s International Journal of Climatology has innovated a novel method to increase the efficiency of generating climate prediction data. The study, co-authored by EarthSystemData & led by UEA’s Climatic Research Unit, describes and demonstrates a new research method that enables daily climate projection data to be produced that can represent a greater range of potential climate futures than could otherwise be achieved. Read more below:
The recent increase in complexity of the mathematical models used to predict Earth’s climate is a double-edged sword: more features can be added to these models, better representing the real world, but running the more complex models becomes expensive — both computationally and in real-money terms.
This expense is compounded since to adequately prepare for the continued heating of Earth, a variety of predictions are needed to represent different possible futures: for example, one set predicting what would happen to the climate system when all nations reduce greenhouse gas emissions; another set to simulate a future in which only some countries do – and so on.
Devising ways to reduce the expense of climate predictions is of critical importance then, especially since information is needed now, to build and prepare for current and future heating.
Certain properties of how the Earth’s climate system responds to global heating, can be exploited to help reduce the cost of assembling the necessary predictions. Fundamental here is the tendency of many local climate parameters to change linearly with the planetary–mean temperature change: For example, temperature at a specific city, Tx, will often change as a scaled function, a, of the global mean temperature change ΔT:
T_x=a∆T
[i]
This is useful since it means that once we know the scaling functions, a, for all points on the planet, we can then decipher the local climate change knowing just the future global temperature change, ΔT.
In essence, we do not need to run the ‘full’ climate model. Instead, we can use a less-complex (i.e. quicker and cheaper) model to establish ΔT for a pool of different future scenarios and then calculate the local climate response according to [i].
This technique, pattern scaling, was innovated in the 1990s, and is frequently used to increase the information available to international and national policy makers , including for the UN-IPCC Assessment Reports, fundamental in forming planning strategies for future climate impacts.
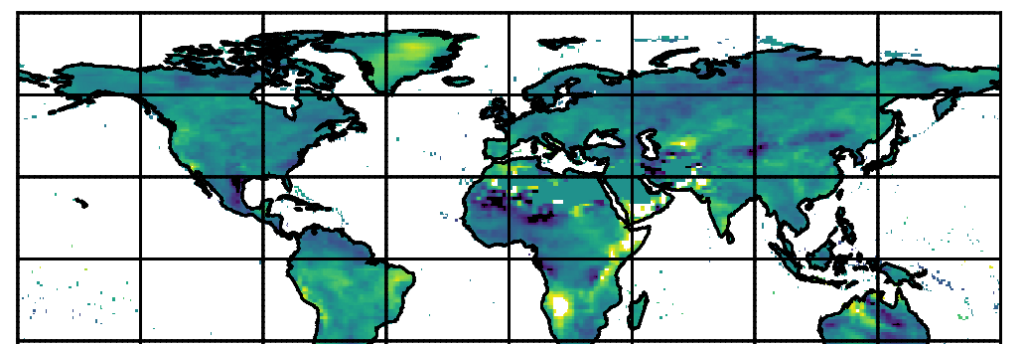
Usually pattern scaling produces monthly-scale data. However, effective climate planning requires information on the daily climate (i.e. the future weather) as well, especially in the case of hydrological planning for floods and droughts. Providing daily climate data can be difficult for complex global climate models – which excel in simulating the slower time-scale planetary processes which modify the climate, but may be less accurate in simulating higher-time scale, and geographically local (i.e. at the city or village scale), data.
For that reason, climate scientists developed separate mathematical tools to blend climate model predictions with statistical properties of local climate taken from real-world weather observations. These tools, via this amalgamation, can produce physically-plausible daily series of data at a very precise geographical location that represents the future climate.
Within this class of tools are so-called stochastic weather generators (bear with me). These generators have a number of statistical parameters which are set to represent the ambient climate in the location they are simulating, and which are then modified to represent the future climate: for example in order to generate a series of plausible rainfall data for a given month, e.g. a March, the generator will need to know characteristics such as: how many days does it usually rain in March at this specific location? What are the chances of a March day being a rain day if the previous one, two, or three days also experienced rain? How much rain might fall in a given day, at this location in March?
To run these tools for a particular future climate, scientists would ordinarily analyse the climate model’s daily data (for that future) to obtain the required statistical parameters for the future, with the remaining set of required statistical parameters being derived from real-world observations.
What if, though, the statistical parameters representing the future could, themselves, be pattern scaled, in a way described above? In other words if you knew, by prior analysis, how each statistical parameter itself responded per degree of planetary warming, then equation [i] could simply be solved – not to predict temperature – but to predict each weather generator setting, knowing only the global-mean temperature change.
Our study outlines the first working framework for this precise combination of techniques — that is the global pattern scaling of weather generator parameters. It demonstrates the validity of the approach using output data from one of the thirty-or-so IPCC class fully-coupled global climate models, IPSL-CM6A-LR, yet the technique can be applied to any climate model that has the necessary diagnostic data.
In short, this means that from just a few sets of training simulations, an untold volume of additional data can be synthesized, approximating any variety of future climate conditions or emission situations not explicitly modelled by the full climate model: the approach enables an extremely cost-effective route to substantially deepening the pool of climate data available to inform climate-impact decision making.
To read the full details of the study you can access the paper online here. This study was performed by lead-author Dr Sarah Wilson Kemsley, as part of her successful PhD research at the University Of East Anglia. EarthSystemData’s Dr Craig Wallace was a co-supervisor, and co-writer to this study, and a co-supervisor for Sarah’s PhD project.